Data’s Pivotal Role in AI: Exploring Its Impact on Financial Services
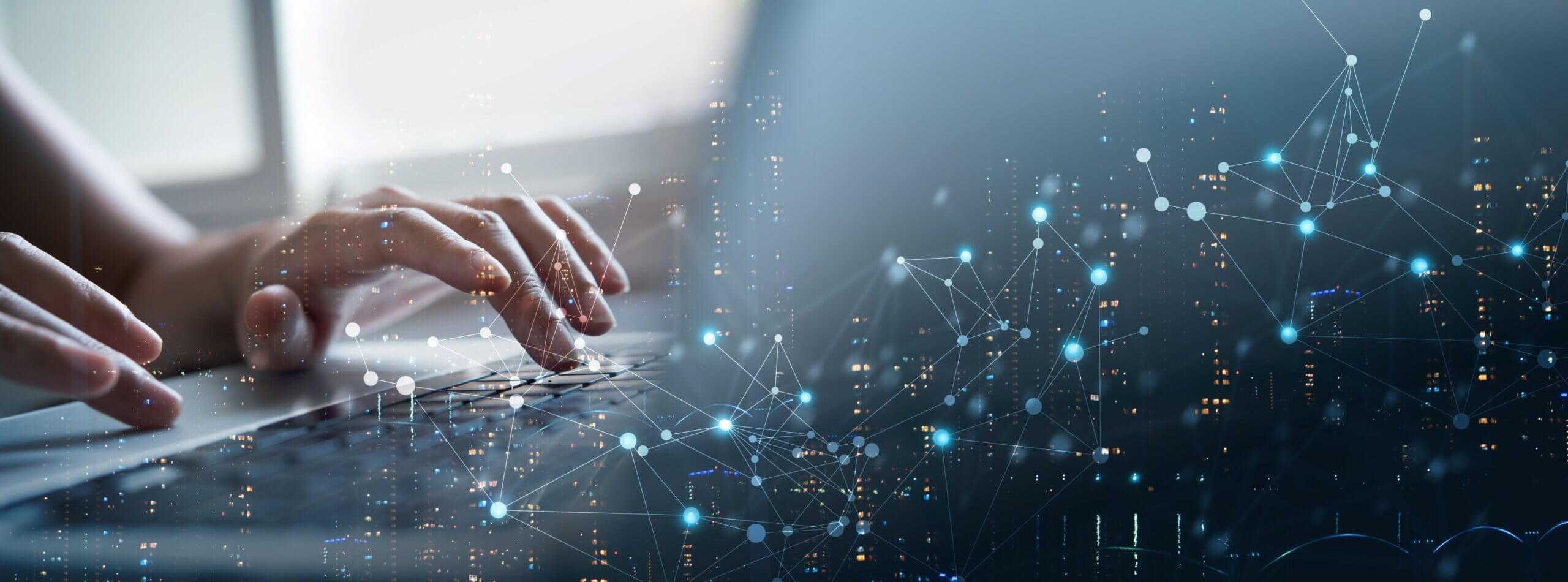
by Joe DeCosmo, Chief Analytics and Technology Officer
The following builds upon a recent VentureBeat article featuring Joe DeCosmo, Chief Analytics and Technology Officer, Enova.
Financial service organizations are leading the way in integrating artificial intelligence (AI) into their operations amid the digital revolution — and the foundation of this transformation is data. To successfully integrate AI, it is crucial to have the right kind of data and to use it correctly.
Solving the Right Problem with AI
Although AI has vast capabilities, it’s important to remember that just because it’s possible to use AI to solve a problem does not always mean it’s the best solution. It’s essential to identify challenges where data can provide actionable insights that are reliable, widely applicable and capable of producing the specific insights needed to address the issue at hand.
Financial institutions can benefit significantly from data-driven algorithms to gain insights into consumer behavior. Using this approach, they can provide personalized financial advice tailored to each customer’s specific financial habits and needs. As a result, customers are more engaged and receive customized solutions that help them achieve their financial goals.
Balancing Opportunities and Risks with AI
Incorporating AI into the financial services industry presents various opportunities and challenges. The potential of AI in this sector is enormous, but it also comes with inherent risks. To strike the right balance and maximize the potential of AI, it is essential to prioritize use cases through a value-based lens. This approach helps organizations to focus their AI initiatives on areas that promise the highest return on investment while mitigating potential pitfalls.
The Essence of the Right Data
The success of any AI project heavily relies on the quality of the data used. Therefore, before embarking on any data-driven solution, it is crucial to have a clear understanding of the business challenge and the use case. It ensures the data used is relevant, unbiased and accurately represents the patterns or behaviors the organization seeks to understand.
Furthermore, it is essential to avoid problems related to poor data quality. Hasty decisions, often made in a rush to deliver results, can lead to undesirable outcomes. It is critical to ensure that data samples cover various dimensions, making the insights derived applicable to the current scenarios and adaptable to future challenges.
Choosing the Right Data Partner
As the adage goes, “Too many cooks spoil the broth.” In the modern world, we could rephrase it as “Too much data clouds judgment.” The overwhelming volume of available data makes discerning quality from quantity even more vital. With the increasing reliance of financial institutions on machine learning and data analytics, the need for accurate, dependable and relevant data has become more critical than ever, and the data source is equally important. Choosing the right partner who can offer reliable and pertinent data becomes paramount, especially in light of the sheer volume of available data.
Moreover, a comprehensive AI strategy requires reliable data partners and an integrated approach that includes AI platforms and fintech collaborators. Due diligence is non-negotiable, given the potential repercussions of a poor choice — especially when dealing with third-party collaborators.
Conclusion
The evolving landscape of AI has thrust data into an even more pivotal role. With continued advancements in AI, the importance of data has significantly increased. In the financial sector, AI is not just a piece of technology. It involves utilizing the right data to tackle the right challenges with the right partners. Financial institutions that master the interplay between data, technology and collaboration best position themselves to lead in this new era.